Smart City and Quartier Mobility
Smart City projects explore ways to make cities more resource-efficient, sustainable, connected, safe, and socially inclusive to counteract urbanization’s adverse effects such as traffic congestion, air pollution, and inefficient resource utilization. Infrastructure-based intelligent sensor systems (ISSs) that collect, generate and provide data play a crucial role in improving mobility. Smart applications typically depend on communicating with such systems, that continuously provides data about their immediate environment and any activity that occurs within it. Urban mobility usually exhibits a characteristic dynamic of its own, which can be understood as a highly dynamic system of complex processes, many of which run concurrently and independently of each other. It helps to look at this system on a smaller scale by focusing on a smaller section that still qualifies as a good representation of the city’s dynamics.
What’s a Quartier?
Quartiers represent an independent social reference system distinguished from others both by geographical and social means. The highest density of interaction is typically located in the infrastructural center of the quartier. Quartier mobility refers to the transportation of people and cargo within its public space with traveling distances less than 3 km.
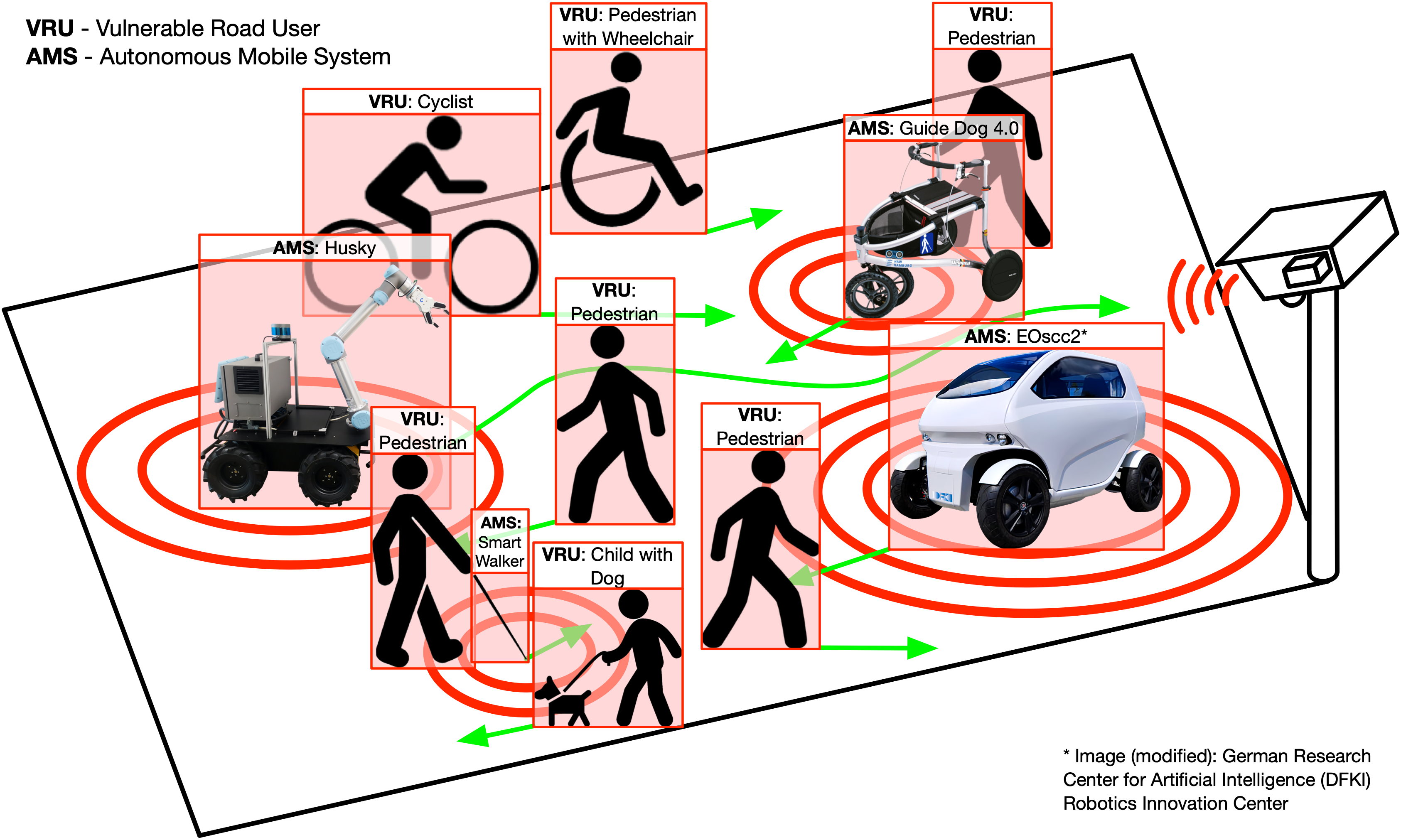
Quartier Mobility Scenario
A quartier can be represented by a residential district, shopping street, pedestrian area, park, school or university campus. Mobility participants such as pedestrians, cyclists, scooter riders, disabled pedestrians or pedestrians with limited mobility are referred to as vulnerable road users (VRUs). A promising approach to optimize mobility is a close network and cooperation between various mobility participants and a dynamic exchange of data with infrastructure-based intelligent sensor systems (ISSs). The usual approach includes data acquisition, exploration and integration with heavy use of AI-based algorithms. Last, acquired and preprocessed data is distributed among mobility participants. The development and evaluation of algorithms for ISSs require vast amounts of data and a test environment.
Project TIQ
The negative impact of global urbanization is most evident in the field of mobility. Inner city individual traffic, in particular, is on the rise. The mixed and increasingly unpredictable traffic conditions result in more and more accidents, to the chagrin of VRUs. The use of existing infrastructures is unbalanced and no longer meets the needs of all road users. By transforming public areas into pedestrian-only zones, a balance is slowly being restored. The deployment of autonomous mobile systems (AMSs) creates new problems that exhibit similarities to those in automotive mobility. Within the project Test Area Intelligent Quartier Mobility (TIQ) at the Hamburg University of Applied Sciences (HAW Hamburg), a multi-sensor system (MSS) is developed. This platform aims to facilitate the integration of AMSs into quartier mobility.
Developing a Multi-Sensor System
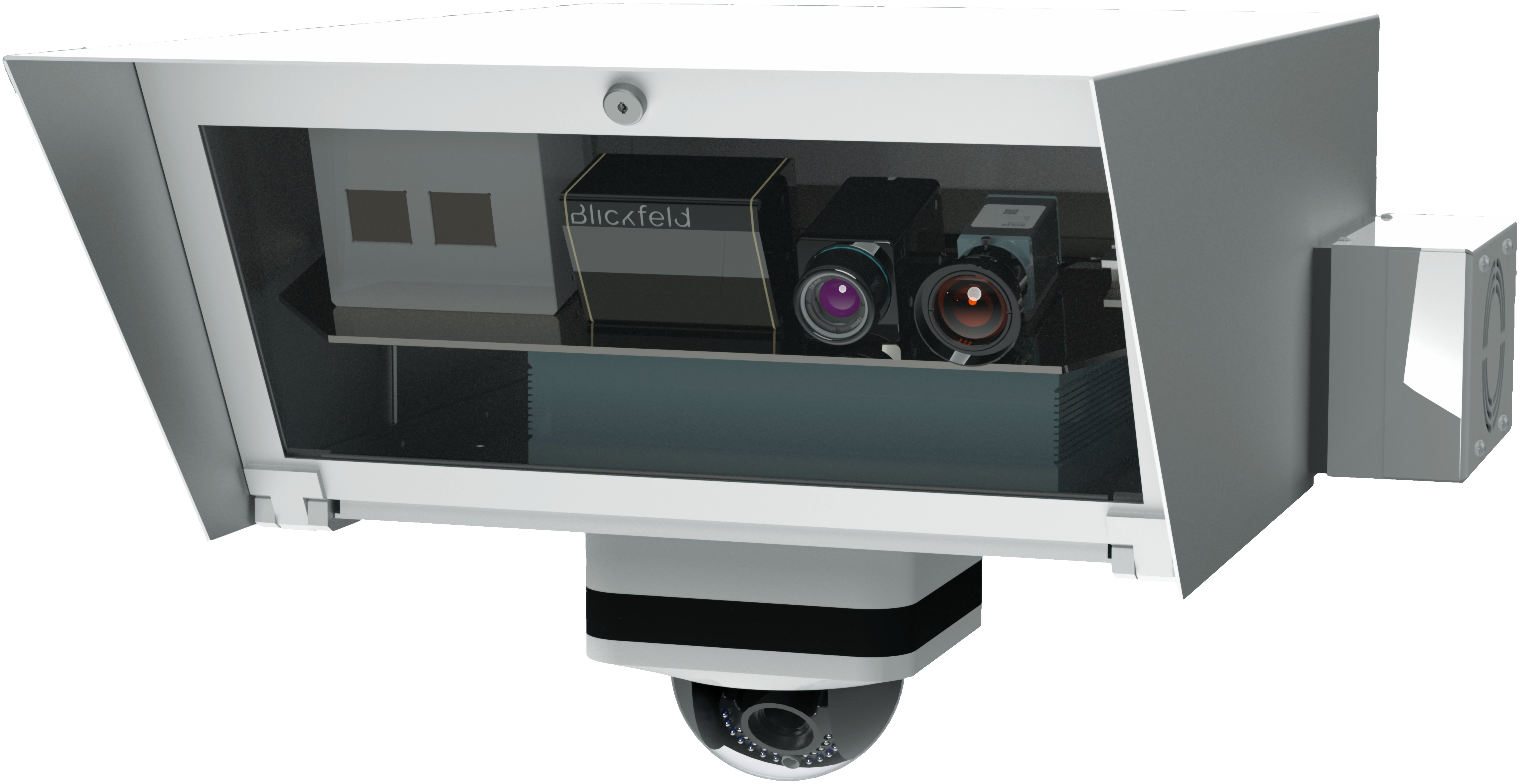
Prototype of the Multi-Sensor System
A MSS is developed to detect mobility events within the test area’s most suitable region using a range of sensors. Methods for detecting and predicting the movements of individual VRUs will be extensively explored. It is worth noting that the sensor’s design and construction specifically focus on Privacy-by-Design to ensure that acquired data does not conclude individuals. Image-based sensor equipment should be avoided in public space since it can be used for identification purposes. To comply with data privacy and protection regulations, installed cameras that are part of the proposed MSS will solely be used for evaluation purposes.

A variety of sensors
Communication between Traffic Participants
In a smart city, all mobility participants continuously exchange data with each other. Depending on the participant from which the data exchange originates, the communication is either called vehicle-to-everything (V2X), infrastructure-to-everything (I2V) or pedestrian-to-everything (P2X). Depending on which road users share data, the communication type is further specified, e.g., the data exchange between vehicles and VRUs (V2P) or MMSs and AMSs (I2V). The same applies the other way around, hence P2V and V2I. Some examples of AMSs which this system is planned to interact with are the Husky, the Shared Guide Dog 4.0, the Smart Walker and the EOscc2 from our partner DFKI. The second and third are aids for pedestrians with physical disabilities and also developed at the HAW Hamburg.
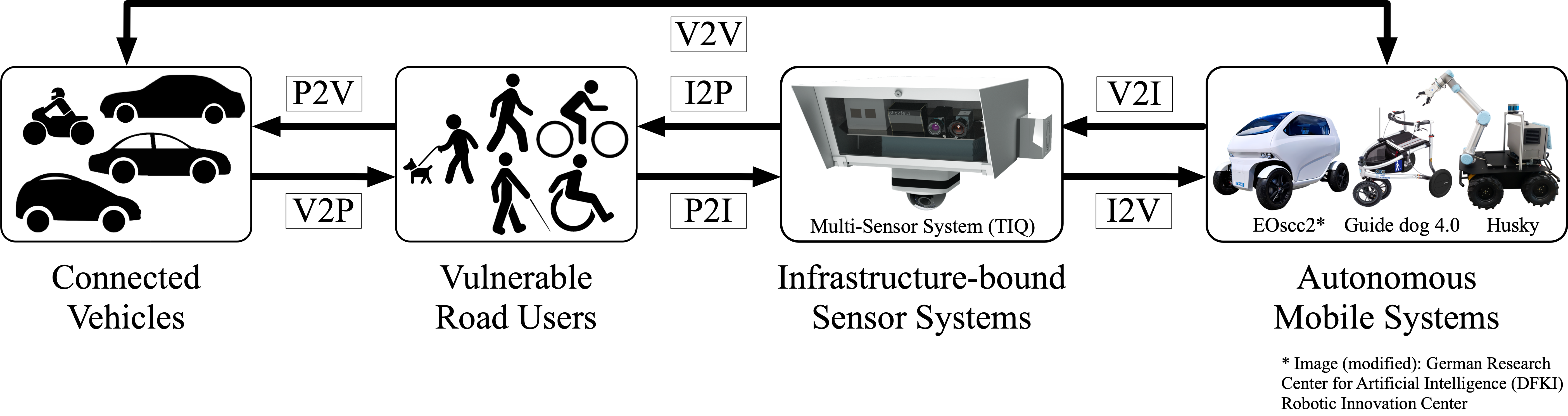
V2X, I2X and P2X Communication
Videos